The added value of machine learning for macroeconomic forecasting in the Netherlands
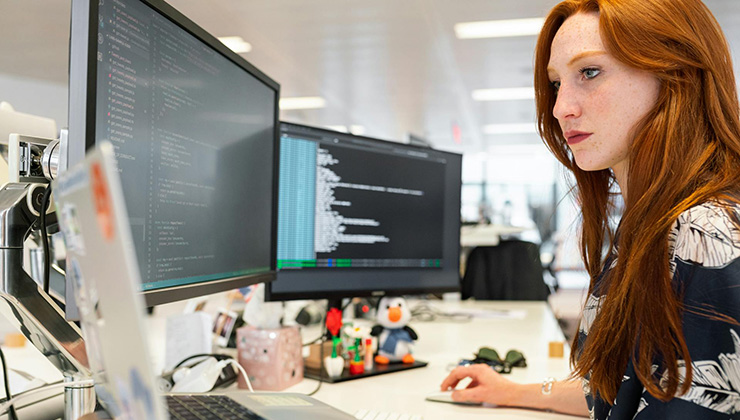
Recent research in the United States has examined which characteristics of machine learning models improve predictive power compared to standard econometric models. However, these results might not directly apply to the Netherlands. To investigate this setting, data from CBS, the ECB, and Yahoo Finance has been combined. We find that the results for the various machine learning features vary significantly depending on the dependent variable. This implies that an approach that is advantageous for predicting one variable is not necessarily advantageous for another variable.
The insights and recommendations described in this study can help Dutch policymakers and forecasters in using machine learning methods for making macroeconomic predictions. However, it is important to realize that machine learning models are still in development. Results found on this dataset are not necessarily transferable to other datasets. Exploring the potential of different models remains necessary when using machine learning.
Downloads
Contacts
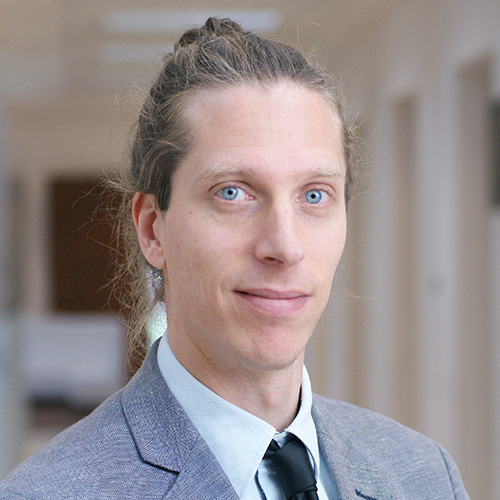